Translating report proportions to incidence proportions
Putting an end to our search for the elusive underreporting factor
Summary
It is my intention to demonstrate how proportional reporting differences in post-approval pharmacovigilance databases like VAERS can be leveraged to establish the side effect profile of a drug.
Today I want to compare several types of adverse events’ incidence proportions found in literature with the report proportions in the VAERS database that include the corresponding MedDRA terms.
Through this exercise we will get an understanding of how incidence proportions relate to report proportions.
We will find that incidence proportions of common symptoms are often identical to their report proportions, while more complex medical concepts are either under- or overrepresented.
Introduction
The report proportion of every MedDRA term is a projection of the respective incidence proportion of the medical concept it describes.
Because the probability for a specific type of event to be reported can not be determined, incidence proportions can not be derived reversely from report proportions.
We can however gather information about incidence proportions from literature and compare those to the report proportions of the respective MedDRA terms in the VAERS database to find out how they relate.
We will look at three examples:
Overrepresented:
Herpes zoster
Equiproportionally represented:
Headache
Underrepresented:
Troponin increased
Methods
Searches in VAERS were performed against the subsample of all US reports which gender field was populated and which age field was either populated by the CDC or parsed by successfully running a simple Regular Expression on the narrative field which the CDC seems to be unwilling to do:
/\W(\d{1,3})\W*ye*a*rs*\W*old$/i
Unlike in my first article, in this one we will be looking at unadjusted proportions.
We will then compare incidence rates from the literature directly to the proportions of the respective terms across VAERS reports.
The results will give us an idea of how the incident distribution translates to the distribution of terms across reports.
Results
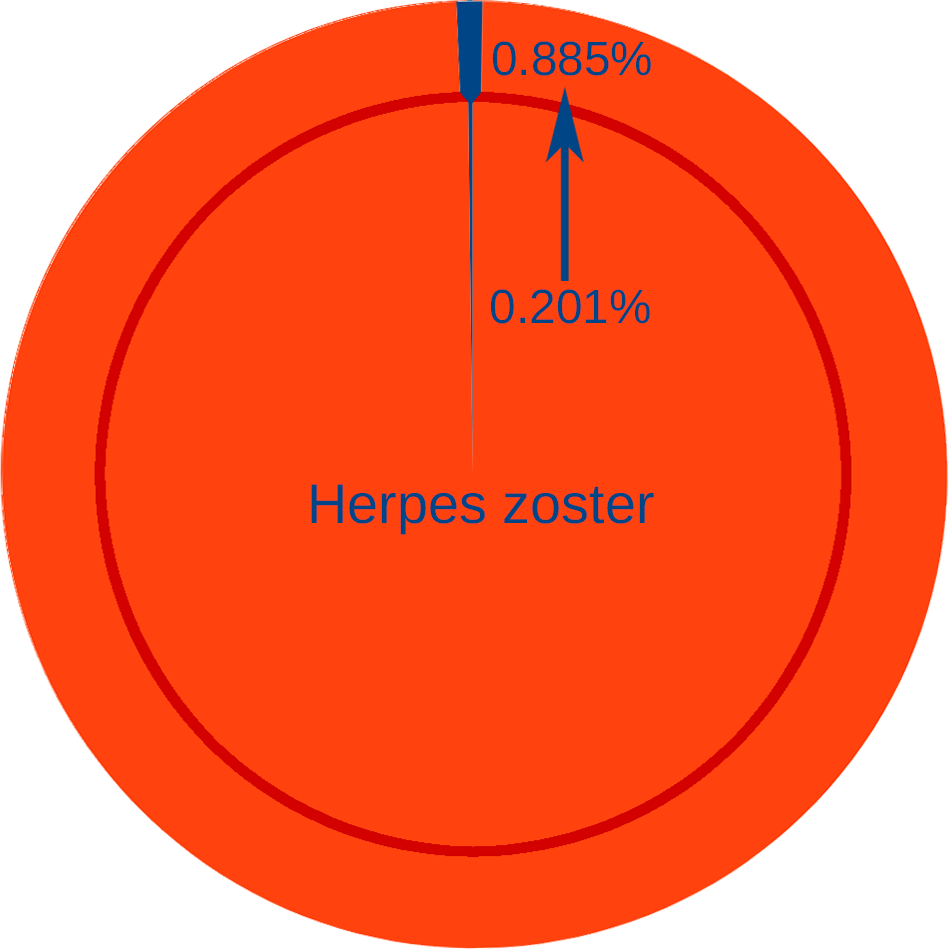
A cohort study from Germany based on real-world data observed 1,095,086 vaccinated patients of which “2204 subjects developed HZ [Herpes Zoster] within 60 days of COVID-19 vaccination”, which puts the risk for Herpes Zoster within that timeframe at 0.201%.
A search in our VAERS subsample for events occuring within 60 days of vaccination against COVID-19 resulted in 678,316 reports of which 6,003 contained the MedDRA term “Herpes zoster” which puts the reported proportion at 0.885%.
“Herpes zoster” is overrepresented by a factor of 4.4. I know very few other such examples.
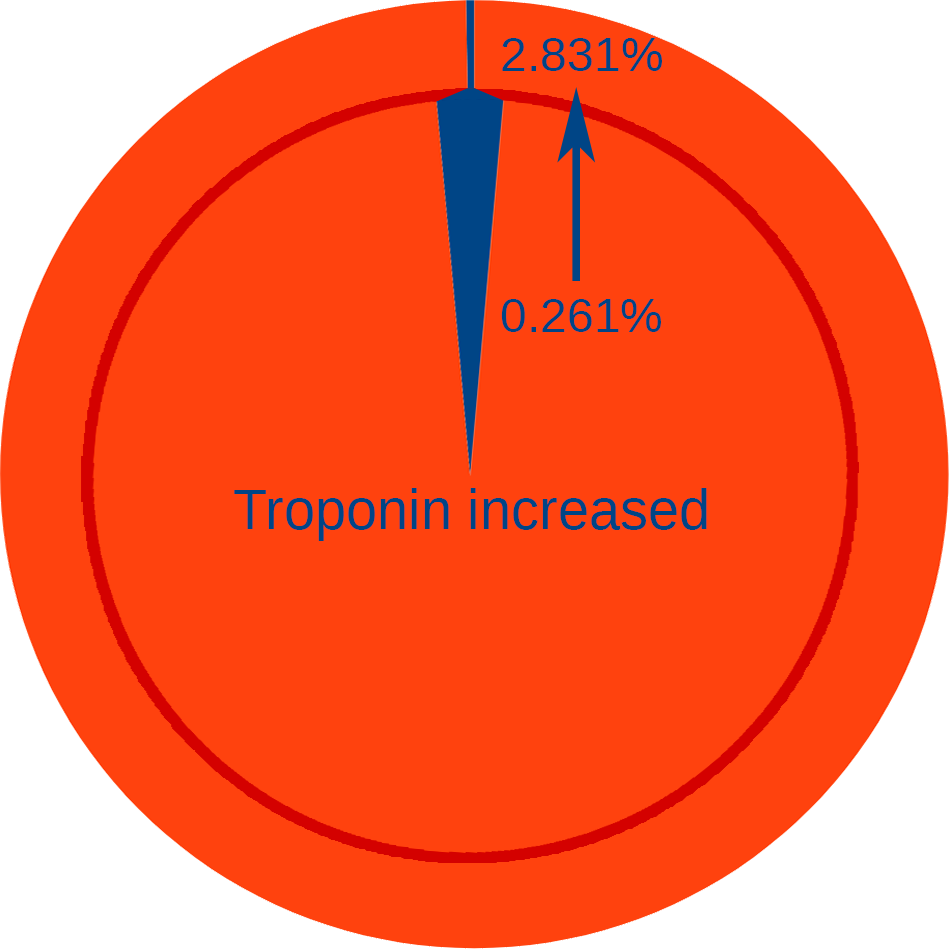
The University Hospital Basel conducted a study on it's own employees to determine to what proportion they suffer cardiac damage after vaccination against COVID-19 by measuring the troponine concentration in their blood. 22 of the 777 employees had elevated troponin on day 3 after their booster dose which means the incidence proportion is 2.831%.
We perform a VAERS search for reports about adverse events that occured within 4 days of a booster dose. 171 out of 65,566 valid reports contained the MedDRA term “Troponin increased” which equals a report rate of 0.261%.
“Troponin increased” is underrepresented by a factor of 10.8. - higher than most.
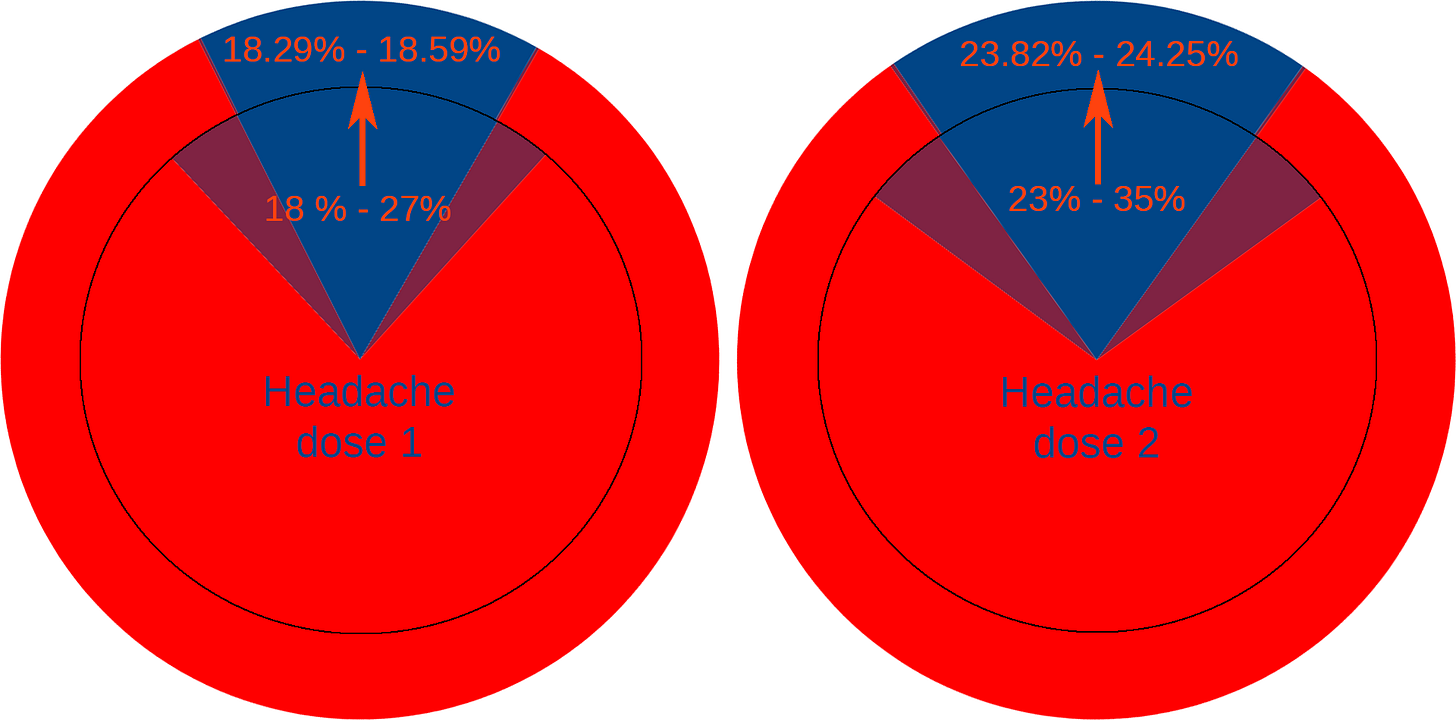
An Italian literature review of 84 papers with a total of 1.57mio patients concluded: “headache is the third most common AE [adverse event] associated to vaccination against SARS-CoV-2, and it occurred in 22% (95% CI: 18–27%) after the first and in 29% (95% CI: 23–35%) after the second dose over a 7-day period”.
A VAERS search for adverse events that occured within 7 days after dose 1 resulted in 45,828 hits for the term “Headache” in 248,526 reports, which comes out to a report proportion of 18.44% (95% CI: 18.29-18.59%). The same search for reports that were submitted after dose 2 returned 37,052 hits across 154,175 reports, which translates to a report proportion of 24.03% (95% CI: 23.82-24.25%).
With our point-estimate within bounds of the Italian review’s 95% confidence interval, we can consider the two values equiproportional.
“Headache” is equiproportionally represented, similar to other symptoms that commonly occur after vaccination against COVID-19 like “Fatigue” or “Pyrexia”.
Note: Pediatric cases below the age of 12 were excluded from the review. Headache’s incidence proportion among the pediatric population is much lower than it is among the adult population. I blame this for the underrepresentation of headaches in the VAERS data (18.44% vs. 22%).
Discussion
When I first realized how close most incidence proportions are to their report proportions, I doubted my sanity, as I so often had these past 2 years.
To understand this witchery, we have to understand why patients seek their doctor and why a report gets filed.
Let’s first look at the 3 types of projections: underproportional, overproportional and equiproportional.
1. When is the report proportion equal to the incidence proportion?
When the occurence of a certain type of side effect has only a minor influence on its probability to be reported. I think the statistics buffs call this effect randomization.
2. When is the report proportion smaller than the incidence proportion?
When a side effect’s chance to be reported is lower than the chance for any adverse event to be reported. Reasons for this can be numerous:
Patient feels self-conscious about symptoms (sexual & psychological side effects)
Patient does not notice the symptoms (laboratory data)
Patient does not associate the symptoms with the administration of the drug (temporal distance)
Misdiagnosis/lack of diagnosis by doctor
3. When is the report proportion larger than the incidence proportion?
When a side effect’s chance to be reported is higher than the chance for any adverse event to be reported. Reasons for this can be:
Extraordinary media attention to a certain type of adverse event as to “Herpes zoster” in the case of the COVID-19 modRNA products
Partilularly severe nature of the adverse event; an example of this is “Death” which is reported by relatives or a person from the treatment team
Conclusion
Equipped with this knowledge, we can proceed to look at reported proportions of myocarditis-associated symptoms in the next article.
The idea is to present an entire system for analysis of post-approval pharmacovigilance data because it looks like we are going to have to get used to to having to deal with this type of thing.
We want to derive a side effects profile - ideally yielding something like a product leaflet for different age groups and genders.
Notes
Before anyone mentions that not every patient experiences adverse events, I will stop you right there. Every patient experiences at least one adverse event: Tissue damage at the injection site.
I offer my code and my help to anyone who needs it. From dataset download over calculating tens of millions of confidence intervals and updating the website to thecustom string decompression and svg generation on the website itself, everything is automated and runs on JS.
If you find the concept I presented interesting, here are a few more terms to check out:
I wonder how they are projected…